AI at the Edge: How Real-Time Computing is Redefining Cloud Strategy in 2025
Research how AI at the edge transforms cloud strategies in 2025 with real-time computing, scalability & business solutions.
The mix of artificial intelligence (AI) and edge computing is changing cloud tech. It brings speed, efficiency, and growth. By 2025, 75% of data will be processed at the edge, not in big data centers. This is for quicker decisions and to save money.
The Rise of Edge Computing
Edge computing is a big change in how we use computers. It moves data processing closer to where data is made. This helps solve problems of old cloud systems, like needing fast decisions and less delay.

Market Growth and Projections
The edge computing market is growing fast. It's expected to hit $61.14 billion by 2028, growing 38.4% from 2021 to 2028. This growth comes from a few main reasons:
1. Proliferation of IoT Devices: The Internet of Things (IoT) is growing fast. By 2025, over 75 billion devices will be connected, making lots of data that needs quick processing.
2. 5G Network Deployment: 5G networks are coming out fast. They offer very low latency and high speed, making new edge apps possible.
3. Demand for Real-Time Analytics: Places like manufacturing, healthcare, and finance need data fast for big decisions. Edge computing helps with this.
Industry Adoption and Use Cases
Many areas are using edge computing to get better and more efficient:
1. Manufacturing: Smart factories use edge computing for quick checks and fixes. This has cut downtime by 25% and boosted OEE by 20%.
2. Healthcare: Hospitals and devices are using edge computing for fast data processing. This is helping with quicker responses and better privacy.
3. Retail: Stores are using edge computing for quick inventory checks and better customer service. This has raised sales by 15% and customer happiness by 12%.
4. Autonomous Vehicles: The car world is using edge computing for fast data processing. This is key for safe and quick driving.
The AI Catalyst: Enhancing Edge Computing Performance

AI and edge computing work together well. AI at the edge, or "edge AI," is changing how we handle data in many places.
Real-Time Decision Making
Edge AI is great for making quick decisions:
Latency Reduction: Edge AI is much faster than cloud systems. This is important for things like self-driving cars, where fast decisions are crucial.
Improved Response Times: In stores, edge AI helps analyze customer actions quickly. This lets stores adjust prices and offers fast, boosting sales by 30% for some.
Bandwidth Optimization
Edge AI also saves a lot of data transmission to the cloud. This means a lot of bandwidth is saved:
Data Reduction: In video surveillance systems, edge AI can reduce data transfer volumes by up to 80% by processing and filtering relevant information locally.
Cost Savings: A mid-sized manufacturing plant implementing edge AI for equipment monitoring can save up to $1.2 million annually in cloud storage and bandwidth costs.
Enhanced Security and Privacy
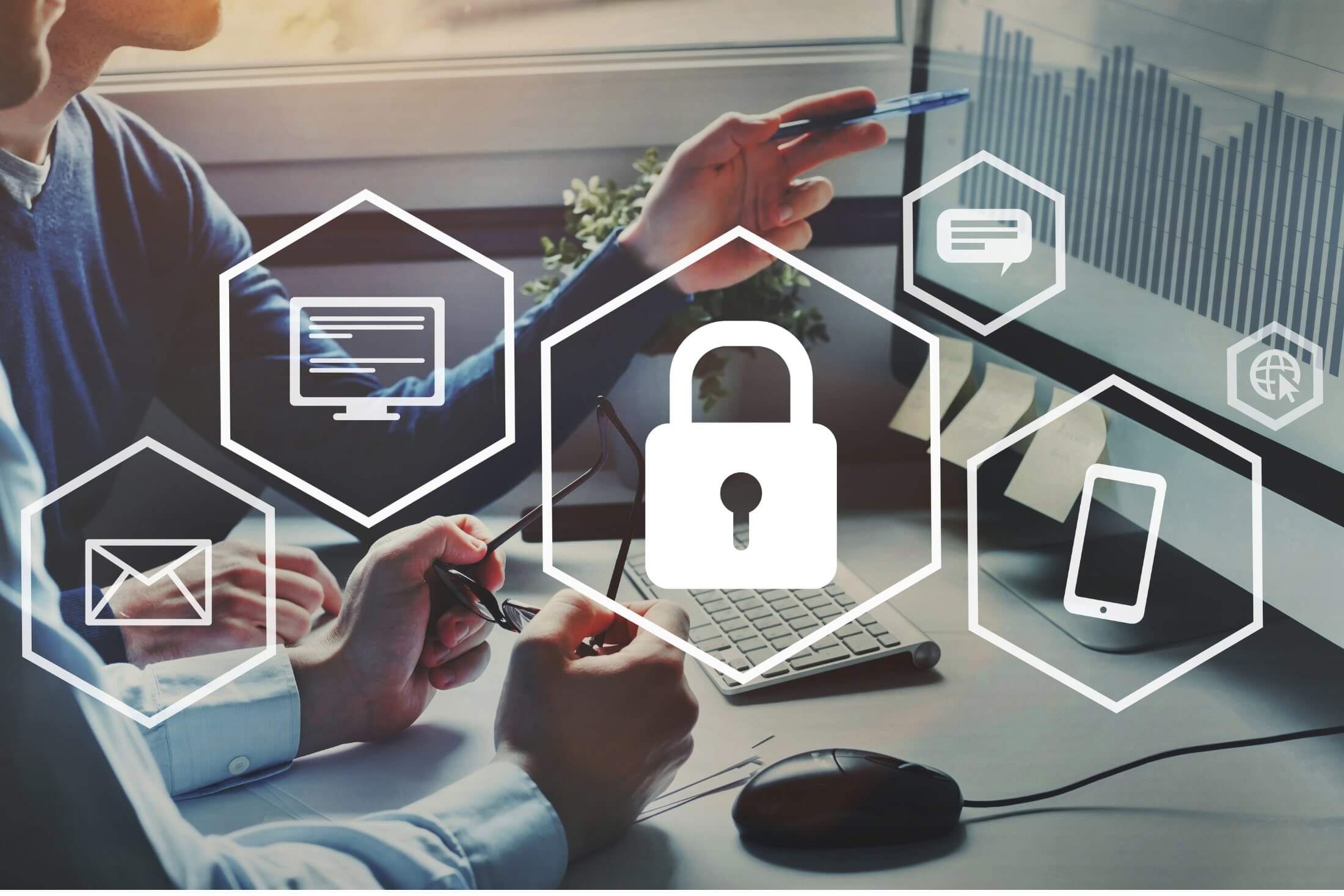
By processing sensitive data locally, edge AI addresses many of the security and privacy concerns associated with cloud computing:
Data Localization: Financial institutions using edge-based fraud detection have reported a 63% reduction in data breach risks.
Compliance: Edge AI helps organizations comply with data protection regulations such as GDPR by minimizing the transfer of personal data across borders.
Hybrid Cloud-Edge Architectures: The Best of Both Worlds
While edge computing excels at real-time processing and local decision-making, the cloud remains indispensable for certain tasks. A hybrid approach that combines the strengths of both edge and cloud computing is emerging as the optimal solution for many organizations.
Cloud's Continued Relevance
The cloud continues to play a crucial role in the following areas:
1. AI Model Training: 78% of enterprises rely on cloud-based GPU clusters for developing and training complex AI models that are later deployed at the edge.
2. Big Data Analytics: The cloud's vast storage and processing capabilities make it ideal for analyzing large datasets and identifying long-term trends. For example, retailers using cloud-based analytics have improved their demand forecasting accuracy by 35%.
3. Global Coordination: Cloud platforms serve as central hubs for coordinating distributed edge nodes and ensuring consistent performance across geographically dispersed locations.
Challenges and Solutions
Despite its immense potential, the widespread adoption of AI-powered edge computing faces several challenges:
1. Infrastructure Complexity
Challenge: Managing and orchestrating thousands of distributed edge nodes presents significant operational challenges.Solution: Kubernetes-based orchestration platforms are emerging as a popular solution for simplifying edge deployment and management. These platforms can reduce deployment times by up to 70% and improve resource utilization by 40%.
2. Security Risks
Challenge: The distributed nature of edge computing expands the attack surface, potentially increasing vulnerability to cyber threats.Solution: Advanced security measures such as federated learning and secure enclaves are being implemented to protect edge AI systems. Federated learning, in particular, allows AI models to be improved without sharing raw data, reducing security risks by up to 50%.
3. Skill Gaps
Challenge: The rapid evolution of edge AI technologies has created a shortage of skilled professionals capable of designing and maintaining these systems.Solution: Low-code and no-code AI platforms are bridging the skill gap by enabling faster development of edge applications. These platforms have been shown to accelerate development times by up to 65% and reduce the need for specialized AI expertise by 40%.
Future Trends (2025-2030)

As we look beyond 2025, several emerging trends are set to shape the future of AI-powered edge computing:
1. Micro AI: TinyML frameworks are making AI possible on tiny devices. By 2030, AI models could be on IoT sensors for just $1. This opens up new ways for intelligence to be everywhere.
2. Edge AI Marketplaces: Edge AI marketplaces will soon be like cloud marketplaces. They will offer pre-trained models and ready-to-use edge apps. This could cut the time it takes to bring new edge AI solutions to market by up to 80%.
3. 5G-Advanced and 6G: Wireless tech will keep getting better, pushing edge computing further. 5G-Advanced and 6G will bring sub-millisecond latencies and terabit speeds. This will enable new edge apps in augmented reality, holographic communications, and swarm robotics.
4. Quantum Edge Computing: As quantum computing grows, we might see quantum-enhanced edge devices. These could solve complex problems in real-time. This could change fields like finance and drug discovery.
Conclusion
We're on the edge of a big tech change. AI-powered edge computing is more than just an update. It's a new way to use data and interact with it. The next few years will be full of innovation and change, with edge AI leading the way in many areas.
FAQ
What is the integration of AI and edge computing in cloud environments?
AI and edge computing work together in cloud environments. This means AI powersedge computing in the cloud. It makes data processing faster and improves decision-making in real-time.
What is the role of AI at the edge?
AI at the edge means AI is on devices or gateways. It makes data processing smart and fast. This reduces the need to send data to the cloud, making systems more responsive.
How does cloud-based AI integration work?
Cloud-based AI uses cloud power to manage AI models. It offers advanced AI capabilities while keeping edge computing benefits. This setup is flexible and fast for certain tasks.
What are the key components of AI-edge integration in cloud environments?
Key components include edge computing, AI model deployment, data management, and security. These parts work together for seamless AI and edge computing in the cloud.
How can edge computing be deployed in the cloud?
Edge computing in the cloud uses cloud services for data processing. It's done by using edge computing services or devices in the cloud. This approach is faster and more efficient.
What are the benefits of edge-AI cloud solutions?
Edge-AI cloud solutions improve efficiency and reduce latency. They also enhance real-time decision-making and save costs. This combination optimizes cloud infrastructure and boosts performance.
What are the technical considerations for implementing AI-powered edge computing?
Technical considerations include edge infrastructure, AI model deployment, data management, and security. Evaluating these factors ensures successful AI and edge computing integration in the cloud.
What are some real-world applications of AI-powered edge computing?
AI-powered edge computing is used in industrial IoT, smart cities, and healthcare. It improves efficiency, reduces costs, and boosts competitiveness in various industries.
Thank you for downloading our guide
Now that you've taken the first step in learning how to transform your business, don't stop there. Contact us today so that together we can take your IT strategy to the next level
Get StartedRelated Posts
Discover the top cybersecurity solutions for New Jersey businesses. Learn how to protect your data with expert advice.
Protect your website from DDoS attacks with our reliable DDoS protection services in New Jersey. Secure your online presence and keep your business running.